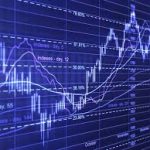
With discussion of systematic strategies becoming more prominent among fund selectors, do people actually know what they are buying into?
In this exclusive piece, financial professional and quantitative investment specialist Elisabetta Basilico looks into the ‘algorithm aversion’ which could be holding back greater investor uptake.
As a quant by training, I often need to explain the big difference between someone like me and a more traditional discretionary investment manager. To me, it comes down to emotions.
Both I and a systematic manager make investment decisions based on rules, evidence and algorithms, whereas the discretionary manager includes his/her opinions and personal judgement – or those of others – into the process.
Despite their recent growth, the reality is that discretionary managers win the battle over investors’ assets. For instance, in the hedge fund space only 31% of the managers are systematic and they manage 26% of the total AuM (Harvey et al., 2017).
Numbers are not much different in the US equity mutual fund space. According to Abis (2017), quantitative equity funds manage 14% of the total US equity mutual fund assets.
Are these numbers justified by risk adjusted performance? An article published in the Journal of Portfolio Management this summer attempts to answer this question.
Campbell Harvey from Duke University and two colleagues analyse “selection and survivorship” bias-free data on 9,000 hedge funds over the period from 1996 to 2014.
By using an algorithm text analysis, they classify the funds into discretionary and systematic and start crunching performance statistics (below is the summary table taken from the article).
Systematic Macro | Discretionary Macro | Systematic Equity | Discretionary Equity | |
Return average | 5.01% | 2.86% | 2.88% | 4.09% |
Return attributed to factors | 0.15% | 1.28% | 1.77% | 2.86% |
Traditional | 2.08% | 1.58% | 1.47% | 2.19% |
Dynamic | 1.28% | 0.98% | 0.23% | 1.08% |
Volatility | -3.21% | -1.28% | 0.07% | -0.41% |
Adjusted return average (alpha) | 4.85% | 1.57% | 1.11% | 1.22% |
Adjusted return volatility | 10.93% | 5.10% | 3.18% | 4.79% |
Adjusted return appraisal ratio | 0.44 | 0.31 | 0.35 | 0.25 |
The risk and factor adjusted performance results (summarised by the appraisal ratio) show that, at minimum – and we are trying to be conservative here – systematic macro and equity funds performed at least as well as the discretionary funds.
Another recent working paper by Simona Abis, a Columbia University researcher, investigates the universe of US equity mutual funds.
She finds, while discretionary managers have greater risk-adjusted performance in recessions, quantitative managers display better portfolio diversification and risk management throughout the business cycle.
Additionally, the author finds that quantitative managers, analysed in her sample, charge 10% lower expense ratios and 9% lower management fees.
Inside aversion
So why aren’t investors buying more “systematic” investment styles? In a recent paper, a trio of scholars from the University of Pennsylvania, talks about a phenomenon called “algorithm aversion”.
The authors performed a series of experiments. They asked participants to predict real outcomes from real data by giving them the possibility to bet on human forecasters or on a statistical model.
The statistical model outperformed the humans in the forecast but nonetheless it is not a perfect tool, meaning it did make some mistakes.
In the different experiments, the participants were first allowed to observe the human forecasts but not the model and vice versa as well as they observed both.
The authors found that, while seeing a model make relatively small mistakes consistently decreased the participants’ confidence in the model, seeing a human make relatively large mistakes did not consistently decrease their confidence in the human.
In general, people are more likely to abandon an algorithm than a human judge for making the same mistake. We tolerate human errors at a higher rate compared to machine errors.
Scientific evidence on the superiority of algorithms and models to make forecasts in many aspects of life is plentiful and it goes back a long way (Meehl, 1954; Dawes, 1979; Silver, 2012)). However, people prefer humans over algorithms.
With our biases, we are precluding superior approaches in tackling many tasks, not just investing. This is a problem for society.